Introduction
The standard gear reducer is an important part of the blender and is also a common and frequently malfunctioning component of the instrument. It has been tested using sensor technology to The shell, gear shaft, input/output shaft, and oil tank of the gearbox collected temperature, vibration, envelope, noise and other information, and extracted the input shaft torque and output shaft torque operating parameters during the operation of the gearbox. It is expected that the operating parameters and working environment parameters of the equipment can be used to predict the upcoming faults. Fault prediction is the core content of industrial Internet applications, while traditional machine learning algorithms such as SVM, RF, GBDT are not ideal for high-dimensional nonlinear data processing. A method for anomaly data detection combining principal component analysis and improved SVDD was proposed. Firstly, the PCA method was used to process the data and extract features from the data with significant impact on detection performance. Then, a classifier was constructed using SVDD to detect anomaly data; The CNN network does not consider the relationship between time series data and results in inaccurate prediction. By mining the degradation law of bearings from operating data, a convolutional neural network (CNN) prediction based on similar feature fusion is proposed The method is based on statistical features and time series correlation to extract similar features. After feature selection, the qualified features are selected for prediction. Experiments have shown that this method can predict the remaining service life of rolling bearings well, but due to insufficient consideration of the context of time series data, there are still deviations in the prediction results. When predicting the temperature of the main bearing of a wind turbine, a Bi-RNN model is established. First, a Gaussian mixture model is used to identify the operating conditions, and then a random forest algorithm is used to model and predict the RNN model, thus achieving early warning of main bearing failure. It is believed that accurate failure prediction can provide support for maintenance decisions, avoid large-scale failures, and minimize economic losses. Therefore, a fault prediction model based on LSTM neural network is proposed, which has strong generalization ability under various working conditions and faults. Although the experiment has achieved good prediction results, the LSTM network has poor classification performance for high-dimensional small-sample data, and is prone to overfitting. The above methods do not combine the failure mechanism and internal operating rules of the equipment, and the analysis of the operating state of the equipment is not sufficient. Therefore, this paper proposes a hybrid prediction analysis model for faults based on standard gear reducers. Through feature extraction and variational modal decomposition of vibration signals, the converted frequency domain feature data and amplitude data are fused according to time series, and finally feature extraction is performed through a self-attention network layer to predict equipment failure.
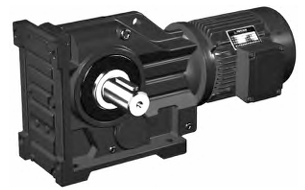
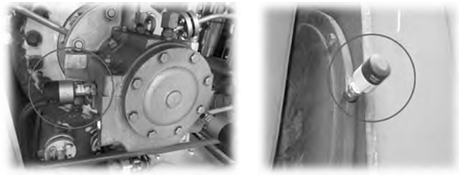
Experimental results and fault diagnosis
The data is divided into three categories: fault data for the month before the fault occurred, data for the four months to one month before the fault occurred, and data for normal operation. The eigenvalues of the three categories of data are used to distinguish between the fault data and the other two categories of data. It can be seen that the signal distribution under faulty operation and normal operation is relatively similar, with only a noticeable difference in expected characteristics. However, the eigenvalues of the six characteristics of the fault data are significantly different from those of the normal operation data. The collected vibration signals are subjected to variational modal decomposition and input into a model for training and prediction. The empirical value of bandwidth limitation is 20,000, which is 1.5 to 2.0 times the length of the sampling point. The number of decomposition modes is 8. The control error constant is 0.001, which determines the accuracy and iteration times. The amplitude-frequency diagram after variational modal decomposition of the collected vibration signals corresponds to amplitudes of 1 to 8 times the frequency from top to bottom. It can be seen that due to the subtle faults in normal operation and faulty operation of the equipment, the collected signals are similar, so the signal amplitude distribution of the two categories of data is roughly the same. However, the fault data is collected after the equipment has failed, and its frequency distribution is significantly different from the other two categories of data. The distribution of fault signals at 1-fold and 8-fold frequencies is significantly different from that of normal signals. Through the above experimental results, it can be concluded that there are significant differences in frequency distribution and iteration times between normal operation and faulty operation of the equipment, and it is possible to determine whether the equipment has failed based on the frequency distribution of the vibration signals over time. The figure shows the visualization of the true value and predicted value for the application of the model in fault prediction. 60 samples are selected for prediction, with the vertical axis representing the category label. 1 represents normal operation data, 2 represents faulty operation data, and 3 represents fault data. The signal distribution of the six characteristics of normal operation data and fault data has significant differences: margin, peak-to-peak value, waveform index, pulse index, skewness, and expectation. After variational modal decomposition of the collected vibration signals, the universality, stability, and continuity are demonstrated. The online test results show that this method is effective and can be used for equipment such as freight cars in the park.
Summary
A hybrid fault prediction analysis model based on standard gear reducers is proposed for equipment operation. By extracting features from vibration signals and performing variational modal decomposition, the transformed frequency domain feature data and amplitude data are fused according to time series. Finally, feature extraction is performed through a self-attention network layer to predict the occurrence of faults. Experiments show that the proposed model can better predict equipment faults and is suitable for fault prediction problems in reducers. However, there is still a problem of data sparsity. If the distribution of various types of data is uniform, the prediction effect will be more accurate.